Is at-home working? Getting to the bottom of how well it's working
The “Operating Model it is a Changing”…
You’ll have to excuse the Bob Dylan reference but the times sure are “a changing”. Almost every business we know is operating in a new state with a significant amount of at-home work. Many transitioned to at-home working in a few hectic days in March. Few had time to plan it properly, but many companies aren’t rushing back to the office. This is partly because the office environment looks unappealing for the foreseeable future with social distancing, closed off areas, restricted lifts and increased concern about employee safety.
Many organisations are clear that at-home work will form a larger part of their operating model even if a vaccine appears. We think that some form of hybrid state with part at-home, part office-based work is the so called “new normal”. Organisational success and in some cases organisational survival will be partly dependent on their ability to design, change and evolve their operating models. They will need to be sustainable, flexible and effective as the business environment continues to alter.

What makes this harder, is that the speed and frequency of change has made it complex to assess what is working and what isn’t.
Every day there seems to be a new study coming out claiming that staff are more or less productive or are missing the office or loving being home. In most businesses, it’s hard to track how productivity is changing because there have been so many factors at play with some temporary and others permanent. For example, often the mix of work has changed, staff are sometimes working longer hours and more digital adoption has kicked in. The situation continues to evolve with secondary lock downs and the novelty of at-home work losing its lustre.
In this paper and its companion (to be published next month) we’re going to look first at how we can get a real handle on evolving productivity for customer facing roles (e.g. calls, emails, administration or processing and chat). Next month we’ll also explore the capabilities needed to manage the work at-home model and consider how to design and implement operating models that can flex to the needs of part at-home, part office work.
Assessing Performance in a Dynamic At-Home World
A multifactorial problem
Measuring productivity as work moved to at-home has been hard because several factors are occurring in parallel such as:
many staff are working longer hours because they don’t have to commute so their output is up but it isn’t a productivity gain, it’s greater availability
absenteeism dropped in the first months of the crisis but has started to return
fear is driving performance as staff are concerned about rising unemployment
the distractions of working in a team environment are gone but…
home schooling has been a barrier and constraint for families at times
connectivity and a new work environment has made it harder for some staff
coaching and quality management are harder in at-home work.
Some of these factors may also have been temporary.
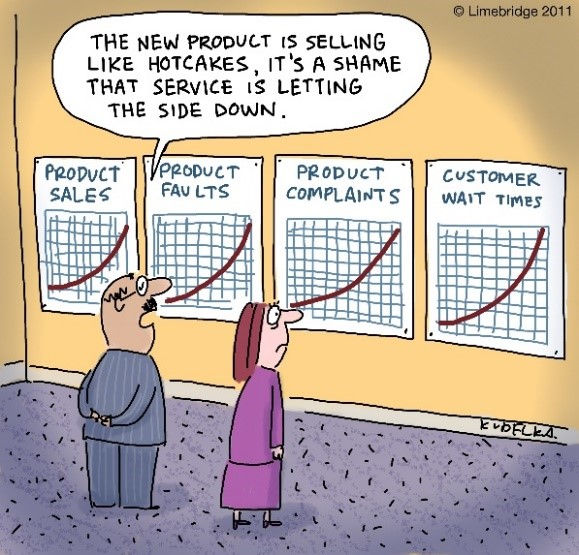
No one is sure if the novelty of at-home work will wear off or whether people will get better at it. The nature and mix of work is changing in many industries and in some cases, who is doing the work has changed which further adds to the confusion. These changes include:
the mix of work has changed as customers have adopted digital solutions at a far greater rate. Greater digital adoption typically means that the complexity of the remaining person-to- person work increases
many organisations have large numbers of unusual or temporary transactions such as mortgage payment freezes or Superannuation withdrawals
other companies have less work because of reduced activity such as insurance claims
companies have redeployed staff between channels e.g. branch staff taking calls
some companies have hired temps while others have downsized
It does appear to be a complex problem. So why is it so important to understand productivity? We think there are four reasons:
There is a risk of burn out and more mental health issues in the current environment so it’s important to separate high utilisation and extended hours from true productivity
Companies want to understand what level of output is sustainable so that they can make correct workforce planning decisions moving forward
If true productivity issues are being masked by other factors e.g. more hours worked, then we need to get to the bottom of the real issues and fix them
The sustainable level of at-home productivity is a key input to the decision making on the extent of at-home versus office work in future business models. Some senior executives believe that no one can be as productive at home and are therefore pushing for a return to work. They will probably only change that view if it’s backed up by hard data and analysis rather than opinion
In essence, having the real facts will help drive more informed decision making.
Multifactorial Solution
One answer to this multifactorial problem is a diagnostic process that uses a combination of “top down” data such as levels of demand and staff utilisation but also analysis of “bottom up” data such as the changing transaction types. We find that the best diagnosis looks at both “demand”, namely what work is now being requested, and “supply”, or the work is being carried out. That is another reason that it needs to combine macro data such as contact volumes and overall utilisation with bottom up observation of how work is being performed and what is happening ‘within the work”.
It is even more important to sample the reality of at-home work today because it is less
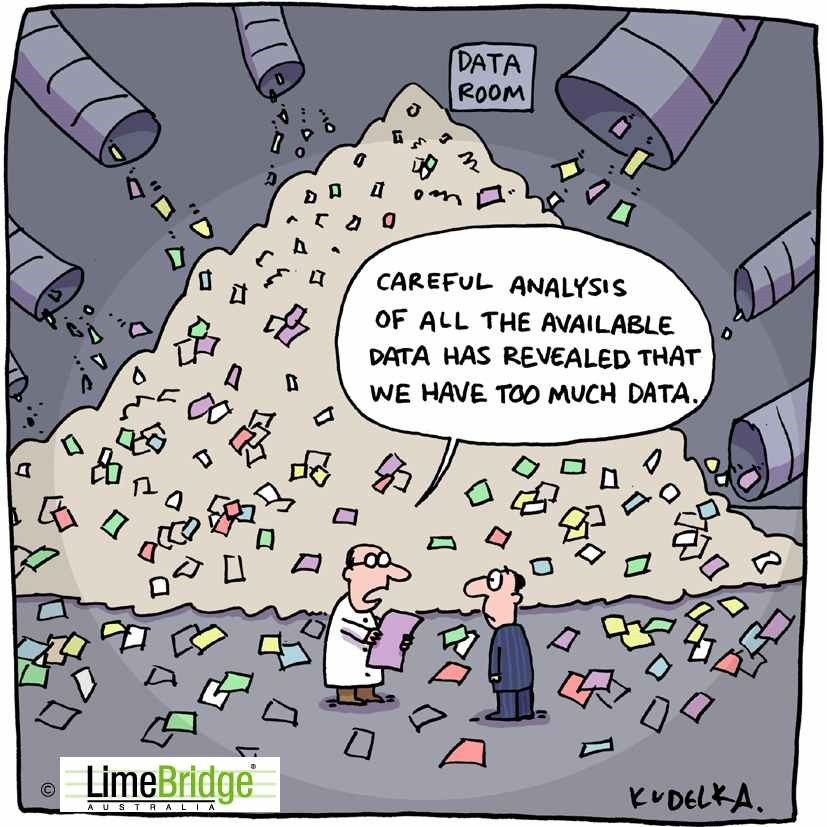
visible. That can involve listening to calls in real time or from recordings, sampling email conversations or auditing processes and outputs. This form of diagnostic uses some of the macro data to establish “what we think is happening” and combines it with bottom up analysis that shows “why this is happening”. The “what is happening” is very important but the “why it’s happening“ helps get to solutions faster.
A recent example demonstrates the difference in these techniques. A company we worked with knew that rates of satisfaction hadn’t changed since at-home work started. They also could see resolution rates remained low. The bottom up observations explained why that was the case. The analysis showed that staff weren’t getting to grips with the customer’s real issues and were rushing hand offs to other areas. This led to more repeat work and even lower customer satisfaction. The organisation has always accepted that the rate of contact was necessary without looking “bottom up” which showed a different story.
Time series is of the essence
It’s trendy at the moment to do one-off data fact slides like this that show “key numbers” in big fonts like this:
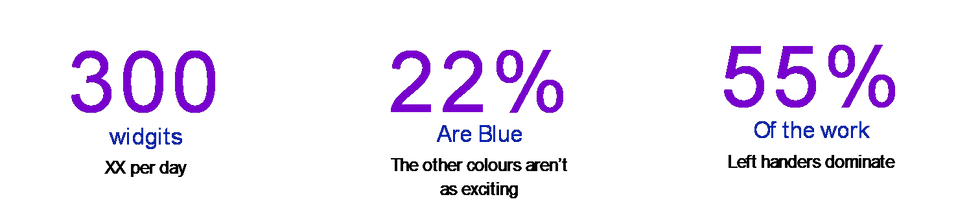
It’s always important to work out what the key numbers are, so we get the idea. However, the COVID-19 crisis is showing how important it is to understand trends and patterns and relationships. We are often surprised how little trend data is reported in organisations or comparisons with equivalent periods (e.g. this time last year or last month). We find that an effective diagnostic needs to combine this macro trend data with the bottom up analysis of why this is occurring now.
In the case of the recent lock downs, where so much changed, so quickly, being able to map the trends and understand what was happening and when, is critical.

This also means looking at the relationship of different data sets. In the example shown, we combined four different time series showing the demand (volume of calls) and supply (staffing levels) compared to plan for each. The time series made key events and issues stand out. The green line, for example, shows that volumes exceeded the plan (the blue lines) but the yellow and black lines (staffing levels) just didn’t keep up. The service level performance made a lot more sense once these trends and relationships were understood.
As there are now so many factors at play, it’s critical to understand and compare the data trends and to think which periods and comparisons are relevant. Proper trend reporting and analysis can really add insight.
Information for Observation: the three-year-old child question
The macro data is critical but it often begs other questions. For example, volumes are up, but why are they up? Handling time is increasing, so why is that? Absenteeism is trending down so why? We’ve been nick-named “the three-year-old child” because of our “why?” obsession. Bottom up observation often provides many of the answers. It provides the detailed supply side analysis that shows what is really happening at the coal face.
In an at-home work situation the coal-face is less visible. That suggests using a range of techniques such as listening to calls live or using recordings, sampling email and chat dialogues in near real time, reviewing complaints or sampling process outputs. Analytics tools can also be applied like automated quality tools or speech analytics samples. However, we do find some manual observation helps direct these tools in better ways. For short sharp analysis, nothing quite beats a human who can analyse many things and draw threads together from a sample of real work. Once the issues are emerging then you can put the AI to work to assess these trends across larger data sets or continuously.
Three examples illustrate the sort of answers that can emerge:
Sampling a series of email dialogues showed that the staff were not questioning the customer or situation well enough before initiating action. On recorded calls the company found the same behaviour and it led to rework and poor resolution. This explained low rates of satisfaction and a high rate of secondary contact.
By sitting all day monitoring supply and demand in an organisation (where staff were at home) it emerged that there were enough people to get the work done but they were being applied to the wrong work at the wrong times of the day. This became even clearer once we helped create a period by period heat map across the different work types (see example above).
Sampling calls showed a company that their staff weren’t educating the customers properly on the available digital solutions even though this was one of the quality criteria. They mentioned the web site, ticked the quality box but not in a way that customers would listen to and often in a way that was rushed and unhelpful.
All three examples illustrate the importance of bottom up observation.
Once an organisation has a better understanding of macro data trends and bottom up observations issues and solutions become far clearer. These techniques can solve the quandary as to whether at-home work is or isn’t working better. Even more importantly, they can unearth solutions to drive improvement.
Summary
In this paper, we’ve shown how detailed diagnosis and analysis is essential to understanding how and why performance is changing in an at-home setting. We know these techniques will help get a grip on the impacts and potential of at-home work. As always, we are happy to discuss these ideas in more detail using the contact details below. In our next paper we’ll describe how to design an effective operating model for part office/part at-home work including the management techniques to be applied.
For more information email us at info@limebridge.com.au or call 03 9499 3550 or 0438 652 396.